Transforming Earth Observation with global AI embeddings
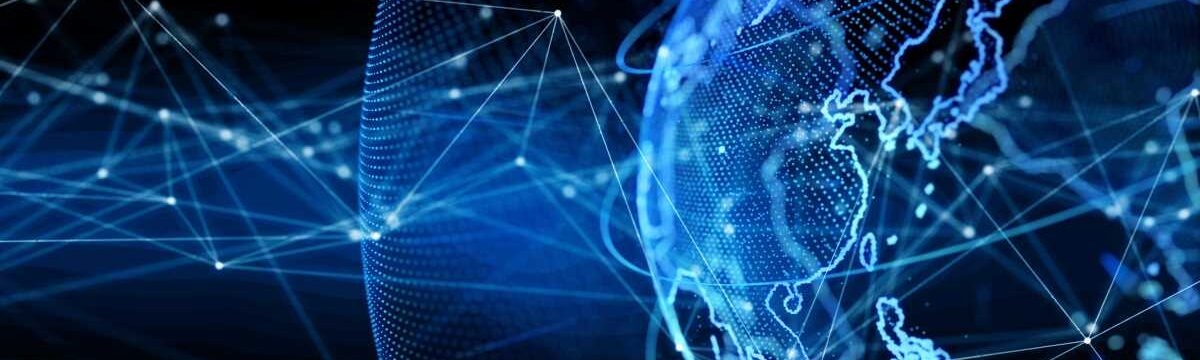
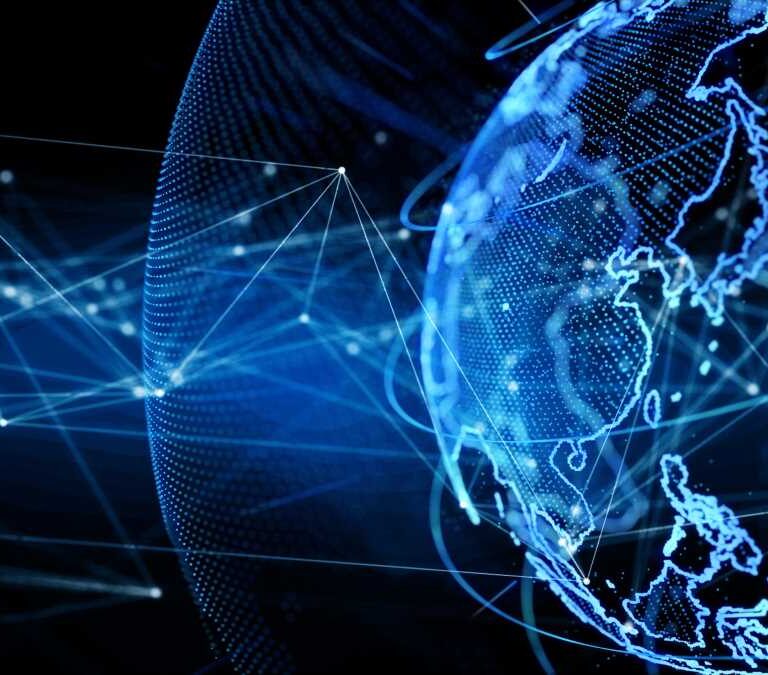
We are proud to announce that as a result of a research collaboration between CloudFerro and Φ-lab, a research laboratory of the European Space Agency (ESA), the first global embedding dataset for Earth Observations (EO) has been introduced. This groundbreaking publication integrates cutting-edge AI technologies to enhance EO capabilities and provide more precise and scalable analysis of satellite data. The AI community can use global embeddings for EO in their research and application development.
Derived from advanced AI models, these embeddings transform vast amounts of satellite imagery into efficient, high-dimensional vector data representations. This innovation marks a significant milestone, enabling smarter and faster analysis of EO data at an unprecedented scale. The global embeddings were computed using the CREODIAS, cloud service platform operated by CloudFerro, powered by GPU-accelerated instances.
“The traditional interaction of users with EO data is going to change dramatically with the wide introduction of embedding products at the scale of full Sentinel data archives. This prototype we built here is the first step towards understanding the value brought by this approach,” says Dr. Mikolaj Czerkawski from ESA Φ lab who led the development of MajorTOM and the technical collaboration with CloudFerro. ”By developing and releasing in a fully open-source setting, we demonstrate how the open data programmes like Copernicus, once again, can deliver unprecedented benefits to the wide community,” adds the expert.
What are embeddings and how do they work?
Embeddings are high-dimensional vectors that transform complex data, such as images or documents into numerical representations. This structured format captures relationships and semantic meaning within the data, allowing AI models to process and analyse it with remarkable context-awareness and precision. This enables machines to identify patterns, similarities, and connections that might otherwise be challenging to detect.
“We’re proud to be at the forefront of such innovation and to realize this ambitious project with ESA AI experts. The Sentinel data embeddings generated with Major TOM and hosted on our CREODIAS platform will bring new capabilities to the geospatial community by making high-quality, AI-ready data accessible globally,” says Dr. Jędrzej Bojanowski, Data Science Manager at CloudFerro. “This collaboration highlights our commitment to embrace the AI revolution and introduce it to EO data ecosystem at large, including Copernicus,” adds the expert.
Embeddings transform raw data into a structured format that can be meaningfully interpreted, allowing AI models to extract deeper insights and relationships. By capturing the underlying patterns and connections within the data, embeddings enable more accurate and context-aware analysis. This approach not only enhances the ability to process complex information but also drives progress in areas such as machine learning, natural language understanding, and computer vision. Embeddings provide the foundation for scalable, versatile AI solutions, unlocking new possibilities across a wide range of applications, from predictive modeling to advanced decision-making systems.
“With this release ESA is adding more momentum to these efforts to help secure a strong position of the European entities in this area,” says Anna Burzykowska, Copernicus Innovation Officer at ESA. “We are keen to continue our collaboration with our industrial and research partners and work diligently to lay the key foundation needed to grow the core of this technology here in Europe, especially for the Copernicus Programme,” adds the expert.
The role of embeddings for EO
Embeddings are increasingly valuable in the field of Earth Observation (EO), offering a range of applications for professionals across this sector. Embeddings can be leveraged by a wide range of professionals across the Earth Observation sector. These include remote sensing scientists, geospatial analysts, and environmental researchers who work with satellite imagery and geospatial data.
How they were calculated
Using Copernicus satellite data, we have generated over 170 million embeddings from 62 TB of raw data, representing 9.368 trillion pixels. By processing more than 8 million images, we condensed this massive amount of information into just 1 TB of optimized data. These streamlined datasets capture essential insights, making it simpler for researchers and analysts to work with the data, fine-tune AI models, and gain valuable insights—without needing to handle the complexity of large, raw datasets.
Available embedding models
This work is part of an expanded standard for releasing Major TOM (https://huggingface.co/Major-TOM) Embedding expansions, now available through open datasets on HuggingFace, including:
- Sentinel-2 Multispectral SSL4EO Model: Core-S2L1C-SSL4EO
- Sentinel-1 RTC SSL4EO Model: Core-S1RTC-SSL4EO
- Sentinel-2 RGB DINOv2 Model: Core-S2RGB-DINOv2
- Sentinel-2 RGB SigLIP Model: Core-S2RGB-SigLIP
Computing environment
Powered by CloudFerro’s GPU-accelerated cloud infrastructure on the CREODIAS platform and guided by ESA’s Φ-lab expertise, this effort demonstrates the potential of AI-driven solutions in EO. The embeddings leverage state-of-the-art vision models like SigLIP, DINOv2, and SSL4EO, unlocking new possibilities for advanced EO tasks.
Plans and development
The next phase involves assessing the performance of these embeddings across diverse Earth Observation (EO) tasks, such as detecting patterns and building predictive models. We will also explore additional foundation models, including MMEarth and DeCUR, to refine their capabilities and ensure seamless integration. Furthermore, the MajorTOM dataset, enriched with embeddings, will be hosted on the CREODIAS repository, providing open access to researchers and fostering collaboration across the EO community.
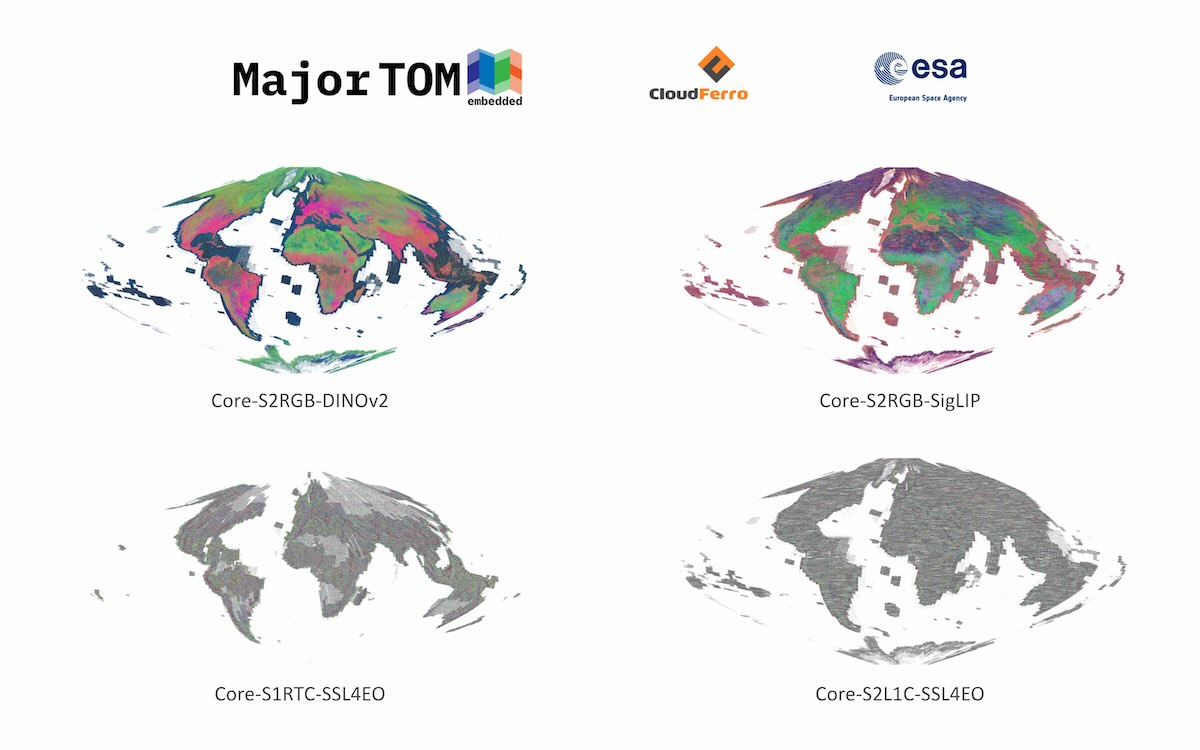