Why Spot instances make cloud computing more sustainable
by Mateusz Ślaski, Sales Support Engineer, CloudFerro
Making any industry sustainable and more environmentally friendly involves minimizing its influence on the entire environment by:
- Reducing environmental degradation by mining or extracting fuels as resources to build power plants.
- Decreasing:
- CO2 emissions
- Water consumption
- Pollution
- Ground coverage by installations.
Power consumption
In the cloud industry, we can optimize power consumption in data centers where cloud infrastructure is located. There are many ways to cut down data centers’ power consumption.
Some important examples are:
- Reduction of losses in power infrastructure thanks to:
- Better efficiency components.
- Shorter power cables.
- Location closer to power plants.
- Improving cooling:
- No mixing of cold and hot air by blanking panels and other ways to force proper airflow through components.
- Proper cable management not to disturb the airflow.
- Using liquid cooling.
- Using controlled variable speed fans.
- Using heat-proof components that allow operating at higher temperatures.
- Air-side and water-side economizers.
- Humidity management.
- Waste heat recovery.
- Hardware optimization:
- Storage tiers (low performance components for less frequently accessed data, high performance components for frequently accessed data).
- Use low-energy consumption components.
- Power consumption monitoring and switching off unused components.
- Consolidate infrastructure and maximize hardware usage over time.
... and many more not mentioned here.
Resources consumption
Building and equipping data centers involves an entire range of technologies that include:
- Ground preparation.
- Building construction.
- Road construction.
- Power infrastructure.
- Telecommunication infrastructure.
- Heating, Ventilation, and Air Conditioning (HVAC).
- Water and sewage installations.
- Computer equipment.
Building Power Plants, including renewable ones, such as wind turbines and solar, involves a similar range of environmental impacts.
Savings
Taking into consideration all these aspects, it seems that an eco-friendly data center would be an installation that involves as few resources and energy as possible, used completely. Then, we focus on the last point "Consolidate infrastructure and maximize hardware usage over time" because:
Fewer physical servers in a data center means:
- No resources and energy consumed to manufacture those devices.
- Reduction of space of the Data Center and resources and energy consumed for DC construction.
- Lower maintenance cost.
- Significant power consumption reduction.
In contrast to other resources, computing power in many cases may be consumed 24/7, therefore many cases of time-consuming calculations may be scheduled and batch-executed outside standard working hours. A smaller data center utilized all the time near its 100% safe capacity in the long term will have a lower environmental impact than a bigger one that is used only partially but requires to be powered 24/7.
Solution – spot instances
However, good will is not enough to change businesses to operate in this way. It is necessary to invest time to identify workloads that can be executed in this way and to develop procedures on how to execute and maintain such calculations. The best way to encourage customers to adopt such practices is to provide significantly lower prices for computing resources.
Here come spot instances as “knights on white horses”. Spot virtual machines are:
- Significantly cheaper.
- Do not lock resources for more demanding customers or critical services.
Spot instances - gains and challenges
- For cloud operators, the gains from spot VMs include:
- Lower investments in hardware.
- Lower cost of server room lease or maintenance.
- More predictable infrastructure load allowing, for example, better agreements with power network operators.
The challenges that cloud operators may face are:
- Lower SPOT prices bring lower income.
- Shorter service windows.
- Single failures may strongly influence customers.
- What customers gain from using spot instances are:
- Significantly lower prices for cloud computing.
- Huge capacity workloads processed in the evenings and nights could be done faster than doing this during working hours with human supervision.
Challenges facing customers are:
- Need to learn to do processing as batch workloads.
- Have to divide own resources into critical ones executed on on-demand instances and those that may be interrupted to run on SPOT instances.
- For everyone and our planet:
Gains:
- Overall less power consumption on running cloud infrastructure.
- Less carbon footprint on infrastructure manufacturing.
- Less natural resource consumption to build server rooms and produce hardware.
Want to learn how to start using spot instances? Read a short guide How to use Spot Virtual Machines.
For more information about spot instances, go to the eCommerce platform. Find out how to test Spot VMs for free.
How to use Spot instances?
by Mateusz Ślaski, Sales Support Engineer, CloudFerro
What are Spot Virtual Machines?
Spot instances are cloud computing resources (virtual machines) available at discount prices. They run using unused provider capacity and may be stopped when the demand for standard-priced resources increases.
CloudFerro implementation of Spot instances
Typical Spot Virtual Machines implementations provide floating prices depending on resources availability. CloudFerro Cloud offers a simplified Spot instances pricing model. Spot instances are available for high-memory, GPU-equipped and ARM CPU-based instances. The prices are flat and not related to changes in availability of resources.
At the time of writing this article, Spot instances prices were:
- 2 times cheaper for GPU-equipped instances
- 3 times cheaper for high-memory instances
- 1.8 times cheaper for ARM-based instances
If cloud resources are available, Spot instances may be launched.
The general documentation about Spot instances usage is “Spot instances on CloudFerro Cloud” https://docs.cloudferro.com/en/latest/cloud/Spot-instances-on-CloudFerro-Cloud.html. It covers important technical details. However, it does not provide more hints on when and how to use Spot instances effectively.
Usage examples
Spot instances are a great choice for:
- Stateless services.
- Short life-span entities.
- Workloads that can quickly dump current data and status when an interruption signal comes.
- Jobs that can be divided into smaller steps executed separately.
Or any combination of the above.
More specific examples are described below.
Test environments with short lifetimes
QA teams often create test environments using IaaS tools, automatically deploy software to be tested execute manual or automated tests, and finally destroy the complete environment. Such test environments have short lifetimes measured in minutes or, at most, hours.
The situation where such tests might be sometimes cancelled due to instance eviction can be acceptable when tests environments costs significantly less.
Additionally, the environment provisioner can be configured so that if Spot instances are not available, on-demand instances will be used for this single test occurrence.
Web services
Most web services can be based on stateless services.
However, you need to plan resources for stable service operation for low and medium load as in case of load spikes. You can configure auto-scaling tools to run on demand instances for low and medium load and attempt running the services necessary for spike handling using Spot instances if they are available. The cost of spike handling is significantly lower. Since service instances dedicated to handling load spikes typically have a short lifespan, the chance of eviction is relatively low.
Another scenario in this area is to go further with this economical approach and configure scaling in a way that on-demand instances are used only for a low constant load range. All instances required to fulfill medium and high load demand would be run on Spot instances. However, with this approach monitoring and eventually replacing evicted Spot instances with on-demand instances would be crucial to assure your service quality.
Batch processing
You can use Spot instances as processing workers if your batch process can be divided into smaller tasks or steps, and the processing workflow is configured in such a way that the entire workflow does not pause or stop in case of stopping or failure of a single step.
The general architecture for such a solution should consist of:
- Persistent storage
This will be the place for all input data, temporary results saved after each calculation step and results. Technically it can be:- An on-demand instance with:
- An NFS server with block storage volume attached.
- A dedicated service or database.
- A bucket in object storage.
- An on-demand instance with:
- Workers on Spot instances.
They will process the work in possibly small sub-batches saving output to persistent storage. - Persistent VM acting as a control node or other work scheduling/coordination solution.
All workers should check the status of tasks to be executed here and report which tasks they are taking to avoid repeated tasks or worse, falling into a deadlock.
This part is not a must. You may design the entire process and prepare workers software in such a way that coordination is performed by them - usually by saving work status on storage. However, a dedicated coordination node would contain additional logic and simplify monitoring status and progress.
Spot Virtual Machines Implementation tips
Resource availability and allocation order
As mentioned at the beginning of this article, Spot instances may be provisioned if cloud contains enough resources for the selected flavor.
If many users started allocating Spot instances resources at the same time, all of them would be treated with the same priority. Instances would be created in an order that requests to the OpenStack API are received.
Speed up instances provisioning by launching them from dedicated image
In the case of Spot instances, fast VM activation is needed. The best practice to achieve this is to
- Install all needed software on a standard VM with the same or smaller flavor than the expected Spot instances flavor.
- Test it.
- Create a snapshot image from the VM.
- Use this image as the source of final Spot instances.
If you do not have much experience with virtual machine images maintenance, you may find it beneficial to learn from available resources and develop your own procedure and tools. I strongly recommend using automation scripts, dedicated tools such as OpenStack Diskimage-Builder, or automation tools like Ansible. Infrastructure as a code tools, as for example Terraform, should be considered.
Below, you will find a simple example of how you can prepare such image using a user-data option when creating the instance.
Please create a new VM using the following command:
openstack server create --image MY_WORKER_IMAGE --flavor spot.hma.large --user-data MY_INSTALL_SCRIPT.sh VM_INSTANCE_NAME
Where MY_INSTALL_SCRIPT.sh contains for example:
#!/bin/sh
apt-get update
apt-get install -y python3 python3-virtualenv
sudo -u eouser bash -c \
'cd /home/eouser && \
virtualenv app_venv && \
source ./app_venv/bin/activate && \
git clone https://github.com/hossainchisty/Photo-Album-App.git && \
cd Photo-Album-App && \
pip install -r requirements.txt'
A VM created in this way will have:
- Python installed.
- An example application.
- Created virtual environment.
- All application requirements installed within the virtual environment.
You can then create an image from this machine by executing the following commands:
openstack server stop VM_INSTANCE_NAME
openstack server image create VM_INSTANCE_NAME --name IMAGE_NAME
The image IMAGE_NAME may be used as a source to create Spot instances with the same or higher resource requirement flavor.
Reacting on an instance termination attempt
The documentation “Spot instances on CloudFerro Cloud” https://docs.cloudferro.com/en/latest/cloud/Spot-instances-on-CloudFerro-Cloud.html mentions that instances can be tagged during their creation or later with callback_url:<url value> tag.
This allows us to react when the OpenStack scheduler tries to delete our Spot instance.
The first reaction option is to convert the instance to an on-demand instance. You need to prepare a persistent service able to receive message about the attempt to delete the instance. Then include the dedicated endpoint’s full address in the tag. When the service receives the instance UIID in message, it should execute script containing the following OpenStack client commands:
openstack server resize --flavor NON_SPOT_FLAVOR VM_INSTANCE_UIID
When output of command:
openstack server list -f value -c ID -c Status | grep VM_INSTANCE_UIID
shows the status VM_INSTANCE_UIID VERIFY_RESIZE,
then the resize may be confirmed with a command:
openstack server resize confirm VM_INSTANCE_UIID
However, we need to consider the following:
- The resize process forces instance to reboot, so if your workload is not prepared, you may lose your data.
- It is not possible to resize this instance back to a spot flavor.
Manual resizing from a spot flavor to on-demand is also possible from the Horizon GUI, but it should be treated as a planned preventive action because humans usually would not have any time to react with the resize after receiving the notification.
If your processing software can save the current state or temporary result, the second reaction option is to place the service on the instance and activate it on a system boot. Then, you need to tag the instance with its own IP address in URL in “callback_url” tag.
When the service on the instance receives a message with information about the instance delete attempt, it may trigger the interruption of the workload and save the current state or temporary result on the persistent storage. In this way, the job can be continued by another still active Spot instance or on an on-demand instance (already active or provisioned if selected number of workers is requested).
Flexible spot instances
When creating Spot instances, you may need to hardcode some configuration data, such as the addresses of input data servers or storage destinations for the results. This can be done using the solution provided in the previous section. However, if you wish to run many different projects, customizing the instance becomes necessary.
A simple functional solution is to:
- Prepare your software to use configuration provided in environment variables
- Inject those variables values thru “cloud-init” when the instance is created
For example, you can create an instance in this way:
openstack server create --image MY_WORKER_IMAGE --flavor spot.hma.large --user-data MY_CONFIG_FILE.yaml VM_INSTANCE_NAME
If your program retrieves data and saves results to REST API endpoints, MY_CONFIG_FILE.yaml could contain:
#cloud-config
runcmd:
- echo "INPUT_DATA_API='https://192.168.1.11/input/'" >> /etc/profile
- echo "OUTPUT_DATA_API='https://192.168.1.11/output/'" >> /etc/profile
- echo "DATA_USER='username'" >> /etc/profile
This file can be generated from a template manually or by a tool/script.
If you are using Terraform, the configuration can be applied using Terraform variables in this way:
#cloud-config
runcmd:
- echo "INPUT_DATA_API='${var.input_data_api}'" >> /etc/profile
- echo "OUTPUT_DATA_API='${var.input_data_api}'" >> /etc/profile
- echo "DATA_USER='username'" >> /etc/profile
Releasing spot instances resources
Using spots is related to the demand for lowering the costs.
If you wish to release resources when the workload is done to minimize the costs even further, then you can consider the following:
- Install and configure a load monitoring tool on the image used to create instances. Check periodically for idle instances, then manually delete idle instances.
- Design and develop notifications about finished tasks into your workload software. Then manually delete the idle instance after receiving a notification.
- Upgrade the previous solution with notifications sent to a software agent working on the persistent VM which can automatically delete idle instances. This solution would be beneficial when the savings from deleting idle instances exceed the cost of maintaining a persistent VM based on a cheap, low-resource flavor.
- Finally, you can build the capability for our software to self-destruct the instance. This can be done in this manner:
- Configure the image with an OpenStack client and credentials.
To do this, follow the steps provided in the documents:
“How to install OpenStackClient for Linux on CloudFerro Cloud” https://docs.cloudferro.com/en/latest/openstackcli/How-to-install-OpenStackClient-for-Linux-on-CloudFerro-Cloud.html
and
“How to generate or use Application Credentials via CLI on CloudFerro Cloud” https://docs.cloudferro.com/en/latest/cloud/How-to-generate-or-use-Application-Credentials-via-CLI-on-CloudFerro-Cloud.html
and information given in the previous chapter “Speed up instances provisioning by launching them from dedicated image”
- Building into your application procedure accessing "http://169.254.169.254/openstack/latest/meta_data.json" to retrieve the instance UUID and then executing self-destruct with command:
openstack server delete INSTANCE_UUID
Kubernetes Autoscaling
We can avoid many of the previous traps by moving to the next level of abstraction: using a Kubernetes cluster created with OpenStack Magnum, featuring autoscaling on Spot instances. With this solution, Spot instances will be used to create Kubernetes cluster nodes when necessary. When the demand for resources decreases, nodes will be automatically removed after a configured autoscaling delay.
As autoscaling and eventual node eviction will be performed on the separated and dedicated node group, then core services on the default-workers node group will not be affected. This solution is cost-effective if the cost of maintaining a working cluster is lower than keeping idle Spot instances. You need to:
- Create a production cluster with 3 control nodes and a minimum one work node. More details can be found in one of the CloudFerro Cloud mini-guides.
- Read documentation about node groups https://docs.cloudferro.com/en/latest/kubernetes/Creating-Additional-Nodegroups-in-Kubernetes-Cluster-on-CloudFerro-Cloud-OpenStack-Magnum.html
- Create a new node-group using one of spot.* flavors.
For example:
openstack coe nodegroup create --min-nodes 1 --max-nodes 3 --node-count 1 --flavor spot.hma.medium --role worker CLUSTER_NAME spot-workers
This command crates a node group named “spot-workers” with a single node activated just after the group creation, and maximum 3 nodes.
Note that explicit definition of all options from the command above is necessary to activate autoscaling on the new group.
Define the affinity of all your persistent services to the node group default-worker.
Follow the example of Kubernetes deployment definition:
apiVersion: v1
kind: Pod
metadata:
name: ubuntu-pod
spec:
affinity:
nodeAffinity:
requiredDuringSchedulingIgnoredDuringExecution:
nodeSelectorTerms:
- matchExpressions:
- key: magnum.openstack.org/nodegroup
operator: In
values:
- default-worker
containers:
- name: ubuntu
image: ubuntu:latest
command: ["sleep", "3600"]
- Define the affinity of worker pods to the node group based on spot instance nodes. Follow the example of Kubernetes deployment definition. Remember to replace “spot-workers” name with your node-group name if you use another one:
apiVersion: apps/v1
kind: Deployment
metadata:
name: counter-log-deployment
labels:
app: counter-log
spec:
replicas: 4
selector:
matchLabels:
app: counter-log
template:
metadata:
labels:
app: counter-log
spec:
affinity:
nodeAffinity:
requiredDuringSchedulingIgnoredDuringExecution:
nodeSelectorTerms:
- matchExpressions:
- key: magnum.openstack.org/nodegroup
operator: In
values:
- spot-workers
containers:
- name: counter-log
image: busybox:1.28
args: [/bin/sh, -c, 'i=0; while true; do echo "$i: $(date) $(hostname) $(uuidgen)-$(uuidgen)"; i=$((i+1)); sleep 1; done']
resources:
requests:
cpu: 200m
Final Thoughts
Using Spot instances certainly brings significant cost savings, but it also provides several other benefits:
- You gain a better understanding of how cloud virtual machine instances work and how to efficiently create, configure, and initialize them.
- IaaC. If you do not use this already, with staring using spots you have opportunity to learn and start to use Infrastructure as a code tools as Terraform.
- Failure (spot eviction) is not an accidental behavior but a feature. Fault tolerance must be a core part of the system design.
- In the case of long-running processes:
- You need to better understand the workflow to split it into smaller chunks that can be processed by SPOT instances. This may provide an opportunity to enable or improve parallel data processing.
- Data structures for checkpoints and temporary data should be analyzed, which may lead to data size optimization. - Adding components dedicated to coordination gives an opportunity to implement detailed monitoring of the entire system’s processing status and progress.
Key benefits of using public cloud computing platform based on open source technologies
By Marcin Kowalski, Product Manager - CloudFerro
Open source technologies are the foundation of many modern IT environments. They can also act as the basis for cloud computing infrastructure, including platforms operating on a great scale.
Open source technologies i.e. based on an open code, allow their users to become independent of many aspects, including geopolitical factors. Such solutions also make it tremendously easier to ensure superior scalability. In this article we present the benefits that open source can bring, and find out why some cloud service providers are betting on open source technologies.
Let us take as an example our cloud computing services, where we build and operate clouds for collecting, storing, sharing, and processing various satellite data on behalf of institutions such as the European Space Agency, the European Organization for the Exploitation of Meteorological Satellites (EUMETSAT), and the European Centre for Medium-Range Weather Forecasts (ECMWF), among others. Every day, we provide thousands of users around the world with access to PB-counted volumes of data delivered by the Sentinel constellation’s European satellites, and a cloud environment designed specifically for their processing.
The nature of the data processed defines significantly the cloud computing architecture. With regards to satellite data, it is crucial to ensure the highest possible availability of both historical and current data (satellite data is available to the user within 15 minutes after it is generated), as well as the scalability and stability of long-term development. This is possible through the use of open source technology and the competences of the provider's team.
Open source as the foundation for cloud computing platform
CloudFerro's cloud platform has been built from scratch using open source technologies as a multitenant environment. We address our services to large European public institutions, and the vast majority of them prefer open-code solutions. From their perspective, open source means the ability to guarantee the continuity of IT environments in the long term.
The ability to scale infrastructure horizontally, i.e. the fact that at some point we will not face a limitation in the ability to expand resources or the ability to support another group of users, is of great importance to the essence of the public cloud model.
From the perspectives of both the provider and the users of cloud environments based on open source solutions, this translates into a number of benefits. Let's summarize the most significant ones.
1. Avoiding the risk of vendor lock-in
In practice, it is important for the supplier, among other things, to ensure the independence of the hardware and application layers. This, in turn, eliminates possible risk of excessive attachment to a single vendor. Over-reliance on one vendor's solutions naturally poses a risk not only to business continuity, but also to the ability to freely set the directions of platform development.
Clients and providers need to make sure that the software we use as a basis for our services, and very often also for our business model, will be available, supported and developed in a long-term perspective. For instance, let’s look at a well-known case of VMware company who has changed its license model, ending the sale of perpetual licenses for their products, and transitioning to a subscription model. This has put many customers in a difficult situation as they have to take into account additional workload and expenses.
The modularity of this technology, that is quite natural for the open source approach, also meets these needs - both in the hardware and software layers. For instance, any component, or even the entire cloud computing technology stack, can be replaced at any time. This gives organizations using such solutions have, among other things, a degree of versatility and the ability of changing easily their cloud service provider. Workloads or data based on standard open source solutions can be directly migrated to another environment providing the same mechanisms. This also translates into new opportunities for ensuring business continuity.
2. High level of technological and operational security
A separate issue is the ability to fine-tune the environments based on open source solutions in line with customers’ or users’ needs. It is of great value that anyone, if they only wish, can thoroughly analyze the application code to fully understand how the entire technology stack works. Openness and technological transparency have impact on the level of data security and user burden.
Statistically, open code technologies do not stand out with a higher scale of vulnerabilities than closed solutions. There are hundreds or thousands of people working on particular solutions within the open source community, so there is a good chance that potential vulnerabilities will be quickly detected and neutralized. The specificity of CloudFerro's cloud services also requires ensuring high resilience and business continuity. This, in turn, is reflected in the framework of internal procedures and regulations implemented by the operator of the cloud.
Last but not least, understanding how the cloud platform components function on a low level, paves the way for implementing unique modifications to tailor its functioning to customers' specific needs.
3. Multi-user accessibility and data isolation of individual users
One thing that differentiates various solutions based on open source technologies is the ability to separate the data and processing used by different users of public cloud environments. This translates into non-obvious technology decisions.
A critical aspect that determines which technology stack will be selected to build public cloud environment is the ability to ensure full multitenancy and complete isolation of individual user's data in a transparent manner. There are a number of technologies that perform well in a single-tenant architecture, but far less well in a multi-tenant architecture. This is especially true in terms of data security issues.
At the same time, as our team’s experience shows, it is possible to build a multi-tenant cloud computing platform that provides full isolation of customer data based entirely on open source technologies. Ensuring end-to-end data separation of individual users of a single cloud environment - including users representing different organizations - is crucial in terms of security, as well as the ability to comply with regulations in certain industries.
4. Cloud platform functional development and adaptation to particular needs
The ability to customize or change the solutions that make up a cloud platform are also beneficial to customers as they can have a much greater impact on setting the development priorities than in case of closed-code solutions.
In the case of closed solutions, their users have a fairly limited influence on the directions of technology or feature development. With open source solutions, the possibilities for determining the vectors of such changes are generally very broad. However, they pose business and technical challenges.
The base for such plans is, obviously, the dialogue between the client and the provider. For many institutions, the very possibility of negotiating the cloud platform development roadmap means greater freedom in defining strategic business plans.
However, in terms of customizing standard capabilities of the open source technology stack, the experience and competence of the team are of great importance, as well as the scale of operations that affects the effectiveness of customization projects.
Naturally, it is unlikely that a single organization is able to implement all ideas on its own, but it can envision at least the direction of the necessary changes. In large-scale organizations like CloudFerro, such a discussion mainly comes down to setting priorities and gradually implementing planned changes. In this sense, open source is much safer and more flexible than closed-code solutions.
5. Freedom to switch suppliers
The greater flexibility of open source technology also means hardware independence. From the vendor's perspective, it is easier to change various elements of the technology stack. For users, it provides greater freedom to migrate workloads to another platform, whether cloud-based or on-premise.
The technology standardization is key here. As the solutions used are technologically similar, they can be more clearly priced, which in effect makes it possible to compare offers of different suppliers. At the same time, technological consistency ensures that the possibility to change supplier is technically feasible with relatively little effort.
In this context, standardization at the level of the technology stack, regardless of possible functional or technological adjustments, leads to into greater universality and versatility in the use of cloud platforms in the face of dynamically changing needs.
6. Price, quality and functional competitiveness
The example of CloudFerro platform shows that the software layer can play a significant role in terms of differentiating cloud platforms in the market. From the providers' perspective, independence in composing the technology stack and freedom of choosing hardware create unique opportunities to compete on price, quality and functional scope of the cloud environment. In turn, from the customers' point of view, these attributes are a significant differentiator between cloud environments based end-to-end on open source solutions and other cloud computing services, particularly those using closed-code technologies. Greater flexibility in terms of cost, quality and functionality, among other things, means the ability to fine-tune the way the entire cloud platform operates to suit unique needs. Significant differences also exist between environments based on open source technologies.
Our customers expect open source solutions. They don't want closed technologies because they are aware of the potential risks associated with them. Instead, they know that open and closed technologies can provide the same values.
Building reliable Earth observation data processing
By Paweł Markowski, IT System Architect and Development Director - CloudFerro
The effective calculations and analysis of Earth Observation data need a substantial allocation of computing resources. To achieve optimal efficiency, we can rely on a cloud infrastructure, such as the one provided by CloudFerro. This infrastructure allows users to spawn robust virtual machines or Kubernetes clusters. Additionally, leveraging this infrastructure in conjunction with tools such as the EO Data Catalog is of utmost significance in accelerating our computational operations. Efficient and well-considered enquiries that we use might help our script speed up the whole process.
Slow count operations on PostgreSQL DB
PostgreSQL database slow counting number of records is a well-known problem that has been described on various articles and official PostgreSQL web pages like: https://wiki.postgresql.org/wiki/Slow_Counting
We will develop an efficient application compatible with the Catalogue API(OData) and highly reliable in handling various error scenarios. These errors may include network-related issues, such as problems with the network connection, encountering limits on the API (e.g., HTTP 429 - Too Many Requests), timeouts, etc.
Workflow implementation
To enhance the IT perspective of this article, we will utilise Temporal.io workflows. Temporal is a programming model that enables the division of specific code logic into actions that can be automatically retried, enhancing productivity and efficiency in the workplace. An intriguing aspect will be the invocation of a Python activity (https://github.com/CloudFerro/temporal-workflow-tutorial/blob/main/activities/count_products_activity.py) from a Golang code (https://github.com/CloudFerro/temporal-workflow-tutorial/blob/main/sample-workflow/main.go.
Now, let us go into the code.
The image below illustrates the schematic representation of the workflow. We have modified the code in order to execute a simple query: count all SENTINEL-2 online products where the observation date is between 2023-03-01 and 2023-08-31
It is important to note that the ODATA API allows users to construct queries with various conditions or even nested structures. Our script assumes basic query structure like:
https://datahub.creodias.eu/odata/v1/Products?$filter=((ContentDate/Start ge 2023-03-01T00:00:00.000Z and ContentDate/Start lt 2023-08-31T23:59:59.999Z) and (Online eq true) and (((((Collection/Name eq ‘SENTINEL-2’))))))&$expand=Attributes&$expand=Assets&$count=True&$orderby=ContentDate/Start asc
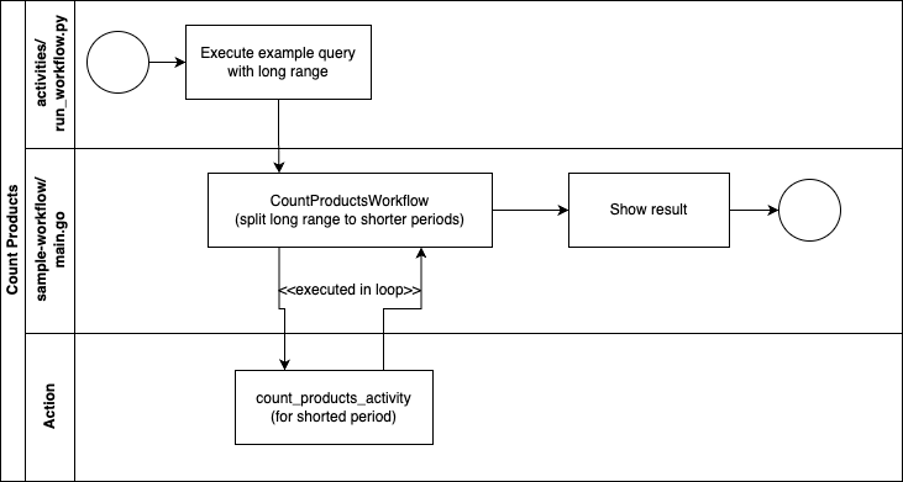
Let’s dive into the technical intricacies of our workflow code, crafted in Go. This code is the backbone of our data management system, designed to streamline queries and drive efficient operations, you can find full source here: https://github.com/CloudFerro/temporal-workflow-tutorial/blob/main/query-workflow/query-workflow.go
At the core of this code lies a remarkably straightforward main function: c, err := client.Dial(client.Options{})
The line above establishes a connection between our application and the Temporal server. If the Temporal server is not accessible, an error message will be displayed.2023/11/07 13:20:50 Failed creating client: failed reaching server: last connection error: connection error
For the comprehensive installation guide, refer to the documentation provided at https://docs.temporal.io/kb/all-the-ways-to-run-a-cluster.
To set your local Temporal server in motion, simply execute the following command within your terminal: temporal server start-dev
With this, your local Temporal server springs into action, awaiting workflow executions.
Once the local Temporal server is up and running, you can activate the worker by executing:
go run main.go
2023/11/07 13:28:30 INFO No logger configured for temporal client.
Created default one.
2023/11/07 13:28:30 Starting worker (ctrl+c to exit)
2023/11/07 13:28:30 INFO Started Worker Namespace default TaskQueue
catalogue-count-queue WorkerID 3114@XXX
Worker implements Workflow that simply divides date ranges into manageable five-day timeframes. This approach ensures that our queries to the EO Catalog will be not only swift but also effective. Armed with these segmented timeframes, we embark on fresh queries, optimizing the process of counting products and advancing toward our ultimate goal.
The optimized process of counting products will help us to get the correct number of specified products.
Run WorkflowWorker: cd sample-workflow/ && go mod tidy && go run main.go
Progress monitoring
In our workflow definition, we have not only segmented dates but also incorporated monitoring functionality, enabling us to query the current state / progress.
currentState := “started”
queryType := “current_state”
err := workflow.SetQueryHandler(ctx, queryType, func() (string, error) {
return currentState, nil
})
if err != nil {
currentState = “failed to register query handler”
return -1, err
}
These queries can be invoked through an API or accessed via the Temporal server Web UI, which is available at the following address: https://localhost:8233/namespaces/default/workflows. You can use the Web UI to check the status, as demonstrated in the image below.
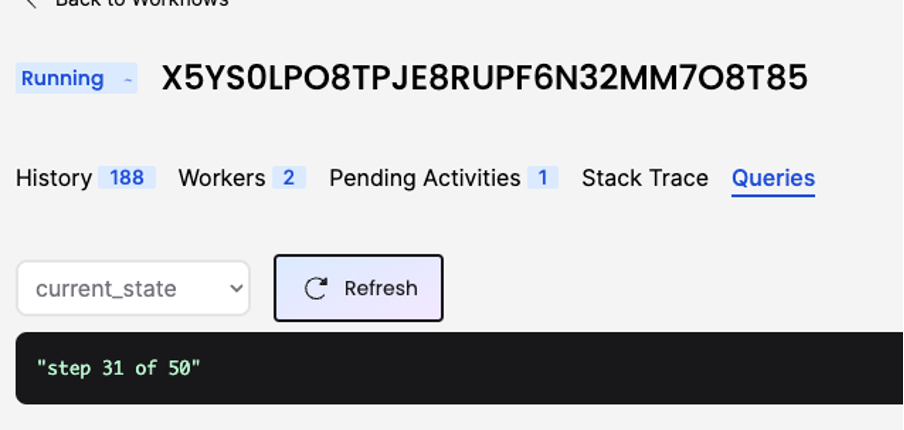
Activity implementation
Last but not least, part of our short demo is the Activity definition.
We can find the code in /activities/count_products_activity.py. That code executes received query with a static 40sec timeout. Before you start running that activity process, please remember about installing requirements.txt in your python env.
A short reminder how to do that:
python3 -m venv activities/.venv
source activities/.venv/bin/activate
pip install -r activities/requirements.txt
Start activity worker: python activities/count_products_activity.py
To execute an example workflow with a sample query, please execute:
python activities/run_workflow.py
CloudFerro’s GitHub repository can be found on: https://github.com/CloudFerro/temporal-workflow-tutorial/
Enhancing Earth Observation capabilities - satellite data applications and implications
By Maciej Myśliwiec, Planet Partners
The view from space offers an expanded perspective on Earth. It is remarkable how data can be obtained and comprehended through satellite-based Earth observation. Sixty-five years after the launch of the first artificial satellite, space technology continues to provide an immense volume of data utilized by analysts and scientists worldwide, enabling the development of solutions across various disciplines. Let us explore the breadth of observations that can be done from space!
Currently, over 4,500 artificial satellites orbit our planet. Among them, there are the satellites of the European Union’s Copernicus programme – the leading provider of satellite data in Europe. Each day Copernicus delivers over 12 terabytes of data that is freely accessible to all users. With a simple internet connection, one can access the extensive collection of satellite images gathered since the programme's inception in 2014, with the launch of the first Sentinel-1A satellite.
Managing and processing such vast amounts of data necessitates substantial computational and storage resources. Earth Observation (EO) platforms like CREODIAS provide users with the means to transform EO data into valuable information. CREODIAS currently houses a repository of over 34 petabytes of data and an integrated cloud computing environment, enabling efficient processing of EO data.
Presently, half a million users of the Copernicus programme process more than 16 terabytes of data daily. To put this into perspective, 1 terabyte equates to approximately 250,000 photos captured by a 12-megapixel camera or 500 hours of high-definition video footage. Hence, we speak of millions of images and substantial quantities of other data (e.g., from satellite sensors) generated within the European programme every single day. The easy accessibility of satellite data fuels innovation across various industries and scientific domains. The current market turnover for Earth observation data is estimated at €2.8 billion, with over 41% of the European industry relying on data obtained from Earth observation programmes.
In recent times, several initiatives have emerged to facilitate data access and processing for scientists, researchers, public administrations, the private sector, and the general public. One of these initiatives is the Copernicus Data Space Ecosystem, which aims to provide comprehensive spatial and temporal coverage of Copernicus EO data, immediately accessible to all users free of charge, along with cloud computing resources for further data processing. Another notable initiative is Destination Earth, the flagship project of the European Commission that strives to develop an advanced digital model of the Earth powered by European supercomputers (HPC) and state-of-the-art artificial intelligence technologies.
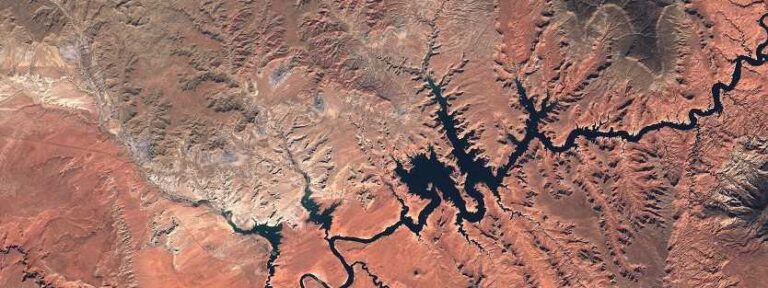
The convenient access to current and historical Earth Observation data enhances planning and decision-making across diverse fields. How can we leverage these vast resources of satellite data?
Environmental research and climate change
One of the primary applications that come to my mind is the study of climate change and meteorology. EO data plays a crucial role in developing meteorological models for weather forecasting, preparing for and mitigating natural disasters, as well as monitoring and addressing climate change.
Accurate knowledge of current land cover and land use is pivotal for effective planning and management by local and regional authorities. Areas under the jurisdiction of public administration institutions, such as national parks, wetlands, lakes, riverbeds, and coastlines, benefit immensely from open satellite data captured by Sentinel-1 and Sentinel-2 satellites. These data offer extensive possibilities for managing such areas with minimal effort. Even 10-meter multispectral data is sufficient for a range of environmentally significant applications, including drought monitoring, flood management, freshwater ecosystem health assessment, forest cover analysis, biodiversity monitoring, air quality monitoring, and land surface temperature analysis.
Sentinel satellite missions, part of the European Copernicus programme, have revolutionized Earth research since 2014. The ubiquitous availability of freely accessible satellite data is complemented by specialized tools that enable their processing and analysis, converting raw data into actionable information.
Illustrative examples of climate change analysis tools include:
- "Global temperature trend monitor": an application that monitors global air temperature changes on Earth's surface over recent decades.
- "Climate monitoring and volcanic eruptions": an application studying volcanic eruptions, which can cause short-term climate effects on a global and regional scale, contributing to natural climate variability.
- "European temperature statistics derived from climate projections": an application providing European temperature statistics for historical periods (1976-2005) and projected 30-year periods (2031-2060 and 2071-2100) under different climate change scenarios.
These applications represent a fraction of the vast array of possibilities available to users of satellite data programs, tailored to address their specific research inquiries.
Forestry
Most forests are managed by state-owned enterprises for timber production, which significantly contributes to national budgets. Effective forestry management requires comprehensive knowledge of soil characteristics, water dynamics, climate conditions, and microclimates across expansive and sometimes challenging-to-access areas. Satellite data offers valuable insights into forest dynamics and their influence on climate patterns. Real-time spaceborne data serves as an invaluable tool for locating forest fires, monitoring plant diseases, and assessing the impact of drought on tree health.
Agriculture
Earth Observation supports various agricultural processes. At the local level, data assists in identifying optimal locations for cultivation, predicting crop yields, and maintaining records of agricultural parcels. Advanced remote sensing techniques, such as Synthetic Aperture Radar (SAR) and hyperspectral analysis, are increasingly being applied in precision agriculture. At regional or national scales, EO data facilitates land cover classification and supports the implementation of programs aimed at agricultural development and direct subsidies for farmers.
Spatial planning
Satellite data plays a vital role in spatial planning for both urban and rural landscapes. Leveraging EO data, one can conduct detailed surveys and complex analyses of areas of interest. Currently, imagery serves as a primary source of information for formulating up-to-date spatial development plans, considering changes in land use and cover, identifying new areas for investment, and detecting wetland areas. Copernicus Sentinel-2 data, for instance, provides rapid and comprehensive insights into current and historical phenomena. Investors seeking to expand their activities in remote regions greatly benefit from information on the status of potential investment areas.
Urban heat islands
Satellite-based Earth observation data also contributes to urban management, including the monitoring and mitigation of urban heat islands, characterized by significantly higher air temperatures within urban areas compared to adjacent rural regions. Unlike traditional point-based air temperature measurements from monitoring stations, satellite data enables the measurement of surface temperatures at any location within a city. This capability facilitates the development of spatial patterns critical for understanding the phenomenon and aids local authorities in taking necessary measures to improve residents' quality of life.
Maritime transport and logistics
Satellite imagery plays a crucial role in the monitoring and detection of vessel traffic on seas and oceans. It also supports the management of maritime economies and ensures the safe transportation of valuable commodities such as liquefied chemicals and crude oil. In the event of accidents, EO images provide timely assistance in mapping the consequences. Combining EO data with Automatic Identification System (AIS) information yields a powerful tool for monitoring marine objects and phenomena, including coastal monitoring and analysis of changing water depths.
Crisis management
Natural disasters like floods, wildfires, and storms often have a wide spatial extent. Open satellite data forms the foundation for the detection and monitoring of such events. Specialized methods, relying on environmental and atmospheric satellite data, are employed to detect favourable conditions and early warning signs of potential natural hazards like droughts or algal blooms in lakes or seas. Synthetic Aperture Radar (SAR) image processing is recommended for monitoring land movement, particularly for applications such as landslide risk detection, vital for mining and mountain tourism. Very high-resolution data plays a crucial role in assessing building disasters, managing mass events, and ensuring the security of government and military facilities.
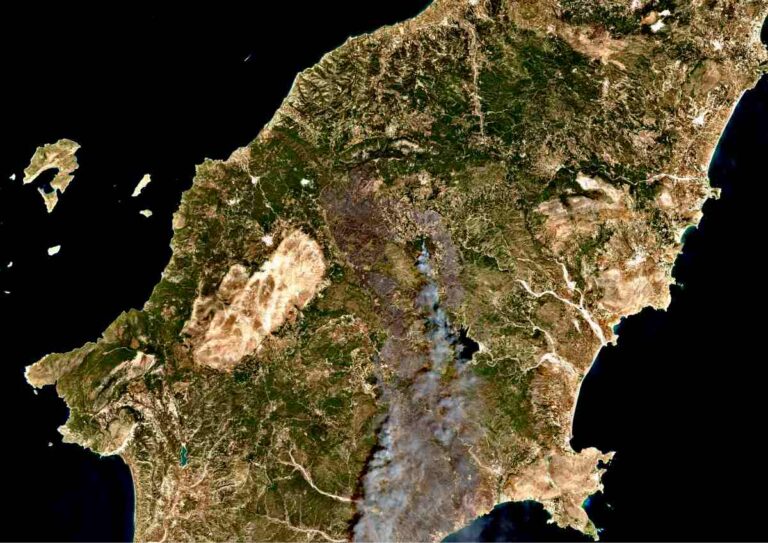
Natural disasters like floods, wildfires, and storms often have a wide spatial extent. Open satellite data forms the foundation for the detection and monitoring of such events. Specialized methods, relying on environmental and atmospheric satellite data, are employed to detect favourable conditions and early warning signs of potential natural hazards like droughts or algal blooms in lakes or seas. Synthetic Aperture Radar (SAR) image processing is recommended for monitoring land movement, particularly for applications such as landslide risk detection, vital for mining and mountain tourism. Very high-resolution data plays a crucial role in assessing building disasters, managing mass events, and ensuring the security of government and military facilities.
Satellite imagery provides an insightful perspective on the locations and magnitudes of flooding events. These visual data enable the identification of inundated regions, assessment of soil and vegetation moisture levels, and the prediction of areas potentially vulnerable to flash floods. Earth Observation platforms like CREODIAS house an extensive array of such images, inclusive of superior-resolution datasets from esteemed programs such as Copernicus. Users have the opportunity to peruse diverse images, categorize them chronologically or geographically, and thus acquire a more profound comprehension of flood dynamics.
When combined with ground-level measurements, satellite-based remote sensing offers an in-depth perspective on global drought trends. Meteorological satellites play a crucial role in monitoring key environmental indicators such as humidity, temperature, and wind, which help predict periods of limited rainfall that can subsequently lead to dry soil conditions and stressed vegetation, commonly referred to as agricultural droughts. To support this analysis, the Copernicus programme provides an array of tools tailored to drought assessment. When it comes to hydrological droughts, which signify water shortages within certain regions, advanced satellite imagery becomes indispensable. One of the standout techniques in this domain is SAR – Synthetic Aperture Radar, which is a type of radar imaging that uses radio waves to capture detailed images of the Earth's surface, making it possible to detect even subtle changes in water levels and providing valuable insights into drought conditions.
Assessment of war-related environmental damage
Satellite data proves invaluable during wars and armed conflicts. However, its utility extends beyond military applications. By processing data, it becomes possible to estimate the scale of environmental destruction or predict actions necessary for post-war reconstruction. An example of such an initiative is EO4UA (Earth Observation for Ukraine), which aims to support Ukrainian institutions and international organizations in assessing the environmental damage caused by war activities within Ukraine. EO4UA activities are conducted in a cloud computing environment integrated with a substantial repository of Earth observation data, encompassing various datasets, such as satellite imagery, crop classifications, forest fire extents, and more, necessary for comprehensive environmental analysis.
The above-mentioned areas provide only a glimpse into the diverse applications of EO data. As we witness rapid technological advancements in space technologies, we can anticipate gaining unprecedented insights into the Earth's ecosystems. We believe that the future will bring a deeper understanding of our planet, facilitated by rapidly evolving satellite data technologies. Equipped with these advancements, we will be better prepared to address environmental challenges on our planet, fostering a more optimistic outlook for the future of Earth.
Direction Earth – European projects in the service of sustainable development
By Michał Bylicki, Sales & Business Development, CloudFerro
Sometimes you can't help but be amazed at the speed of technology development. New tools improve our efficiency, help solve problems, and facilitate everyday activities at work and in play. This is largely due to the availability of increasingly larger data sets and increasingly powerful computing resources to process them.
However, new technologies have an impact on the natural environment, climate, and humans. While generating undoubted benefits, they also carry certain threats. Therefore, it is important that the development of new technologies supports human health and safety and serves, or at least does not harm, the natural environment.
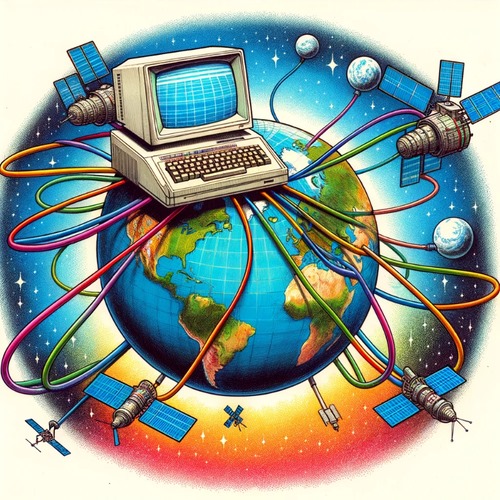
Many companies take into account social and environmental factors and costs in their activities, which, from the point of view of society, are more important than the profits of an individual company. However, this is not always enough, which is why this process is supported at the European and national levels.
One method is to limit undesirable activities through appropriate legislation (e.g., limiting emissions or prohibiting polluting activities). Another way is to introduce initiatives that promote green technological transformation. In Europe, such initiatives include the European Green Deal, Digital Europe, and the European Strategy for Data.
These initiatives involve developing competencies and services in Europe, taking into account the principles of sustainable development. The European Green Deal assumes achieving climate neutrality by 2050, reducing emissions by at least 55% by 2030, decoupling economic growth from fossil fuel consumption, and a just transition. Digital Transformation aims to achieve digital and technological sovereignty in Europe, digitise industry, and ensure access to data while assuring its protection and fair competition. As part of its data strategy, the European Union aims to create a European data market.
One of the most interesting projects related to the above-mentioned initiatives is Destination Earth (DestinE), to create a digital replica of the Earth to model all processes observable on Earth in various ecosystems (e.g. atmospheric and climatic phenomena, forest ecosystems, glaciers, agricultural monitoring and others).
The DestinE initiative consists of several components and is implemented by ESA, ECMWF, and EUMETSAT in collaboration with industry. It is based on data from various sources and very high computing power (HPC and cloud computing). To facilitate the availability and effective use of data, a Data Lake infrastructure has been created to store, process and share data needed for Digital Twin Earth (DTE) processing. The initiative also uses Earth observation data, including data from the Copernicus programme, one of the largest open data sources (available to anyone at no charge through the Copernicus Data Space Ecosystem).
The combination of open access to data with computing power and convenient tools for processing allows companies and institutions dealing with climate monitoring and nature protection to analyse air, water, and soil pollution effectively. It also helps monitor natural hazards such as floods, earthquakes, and fires, supporting prompt action in the event of disasters.
Of course, increased data processing also means higher energy consumption, which is why optimising data processing and storage is even more critical. In this case, using cloud resources turns out to be more beneficial.
Firstly, most cloud providers use renewable energy sources whenever possible and optimise energy consumption. Secondly, using shared resources increases resource utilisation and avoids maintaining and powering unused resources. Thirdly, in the case of demand for Peta bytes of data, processing nearby data is much more effective than transferring it, e.g. to local infrastructure and keeping a local copy of the data.
Ambitious projects, such as DestinE, stimulate technological development, taking into account the principles of sustainable development. They enable observation, more detailed examination of natural processes, and reflection of processes in the real world. They help transform data into information. This way, they increase our knowledge of the world and help us make informed decisions.
At CloudFerro, we contribute to achieving European environmental and technological goals because:
- One copy of data serves thousands of users, a processing chain is more effective in the cloud.
- The use of satellite data enables quick response to phenomena that threaten the natural environment.
- Immediate access to data allows for quick reactions to crisis situations.
- Access to historical and global data allows the observation of trends and comparing them across periods and locations.
- We use and contribute to Open Source technologies, develop competencies locally, and ensure digital sovereignty in Europe and fair competition in the cloud services market.
- We power our clouds with renewable energy sources and optimise processing chains.
- We do not violate the privacy and property of user data.
Lessons learned from my Kubernetes Journey
By Paweł Turkowski, Product Manager at CloudFerro
At CloudFerro, one of our guiding principles is Technological Courage. This value has strongly resonated with me from the start of my journey at the company. It is a simple yet powerful statement that gives our team a clear guideline not to be afraid to operate outside our comfort zone.
Kubernetes, by today’s standards, is not a new technology anymore. It has graduated to being an often primary go-to model of application deployment. CloudFerro was quick to jump on the Kubernetes bandwagon. We have multiple applications running on Kubernetes, as well as a massive amount of K8S jobs running daily to process data in our satellite image repositories.
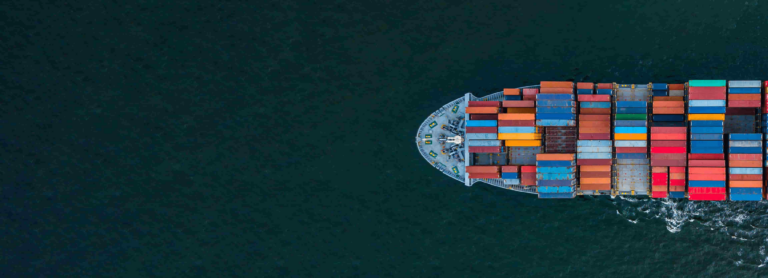
For me, personally, it has been an exciting challenge to be a Product Manager for the Kubernetes product offering. Apart from Kubernetes, there is also a large ecosystem of other technologies required to navigate in the DevOps space. I have worked in the Product Management field for several years, managing a variety of different products. The generic understanding of operating principles is a very useful and transferable skill. Yet I think that taking time to understand the domain well is one of the best investments one can make as a PM.
Some of our clients have been running Kubernetes clusters on CloudFerro clouds for likely as long as this technology has lasted. However, in the past couple of years, we have made some significant commitments to grow our Kubernetes offering as a natively available solution on our clouds.
With OpenStack Magnum, we enable our customers to deploy a functional Kubernetes cluster in literally a couple of minutes. It is a matter of selecting a few options from the Horizon UI. In fact, the only key decision before deploying pods/applications/workloads is to choose your cluster size and underlying Virtual Machine flavors. This setting can also be changed after cluster creation and one can opt for an auto-scalable number of nodes.
Aside from making sure the Kubernetes clusters are quick to set up and reliable to run, we also constantly scan the interaction with various integrations used by our partners, to make sure they work seamlessly with Magnum.
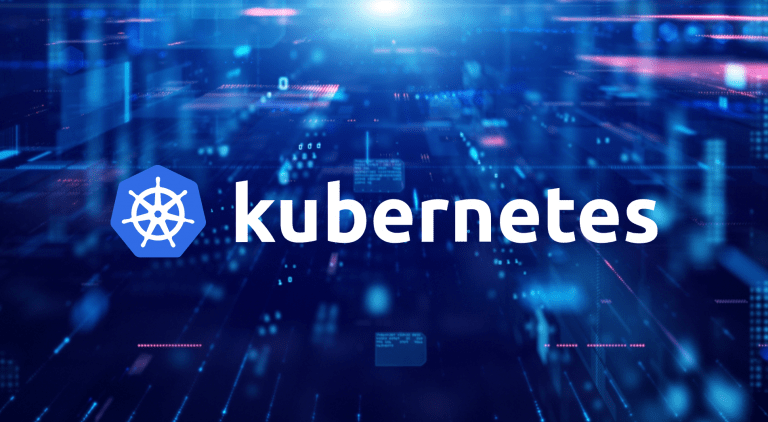
Working with Kubernetes is an exciting journey, where you never stop learning. Some of the lessons I can share from my own perspective:
- Browsing through the documentation for hours might not be unusual for a developer, but it is in fact quite the same for a PM working on a highly technical product. These days there is a lot of high-quality, free supportive content that helps a lot. Recently, Chat GPT is also enabling us all to make some significant shortcuts in various domains, and Kubernetes is no exception.
- Learning from others is valuable, however, it helps to do your own research before asking a question (this refers to colleagues, but also e.g., to online forums). You might be able to answer your own question faster, or, at minimum, narrow down the question to the actual core.
- Speaking to clients is of tremendous value. It helps to get a proper understanding of what features we think might help, and which ones actually move the needle for our partners.
It has been a rewarding experience to see the growth of Magnum and the trust of our clients to run their Kubernetes clusters on the CloudFerro infrastructure. I would like to take the opportunity to invite readers of this post to watch my Kubernetes webinar. It can provide some help to quickly get started with Magnum and Kubernetes overall, with some examples from the Earth Observation domain.
Paweł Turkowski
Paweł has over 15 years of experience in product management. At CloudFerro, he is responsible for the infrastructure offering, currently focusing on the Kubernetes platform.
Copernicus Data Space Ecosystem explained
CloudFerro, together with its partners, has been selected by ESA as an industrial team to implement and operate Copernicus Data Space Ecosystem. The goal is to provide full spatial and temporal Copernicus EO data coverage available immediately and free for the users.
On Tuesday, 24th January 2022, the new Copernicus Data Space Ecosystem was launched. The service is taking the European Union Copernicus programme to the next level, ensuring the data make the greatest possible impact on users, all the citizens, and – ultimately – on our planet. The new Copernicus Data Space Ecosystem builds on existing data distribution services (incl. CREODIAS and others), ensuring their continuity and introducing significant improvements.
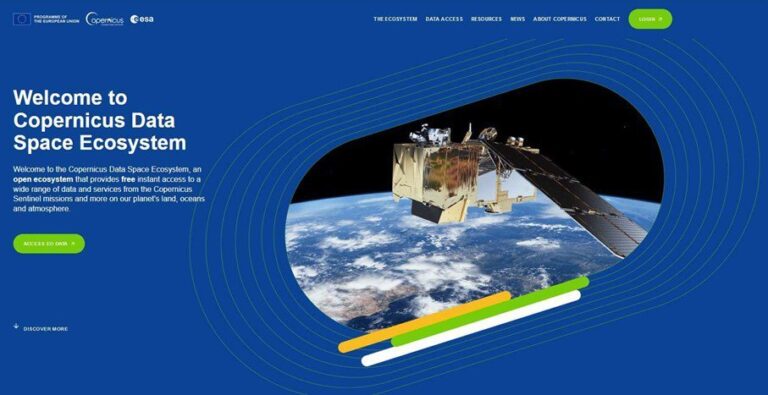
Evolution of Copernicus data access
The European Union Copernicus programme is among the biggest providers of Earth Observation data in the world and its launch was one of the most important events in the remote sensing community over the past decade. With systematic monitoring over large areas, good quality of data, resolution fitting its objectives, ensured the longevity of the program, and, most importantly, a clear and simple open data policy it took the world by storm. This, however, is not something to be taken for granted. When Copernicus started, remote sensing was a niche field occupying mostly researchers and the intelligence community. There was no specific reason to expect that it will be any different with Sentinel data. But it happened.
The data was picked by enthusiasts, then by companies and institutions. Fast forward a couple of years and suddenly there are hundreds of applications helping farmers to better manage their fields, financial markets forecast prices of corn, journalists regularly use it to observe or validate news, the European Common Agriculture Policy relies on its data to monitor practically all agriculture fields for sustainable agriculture practices, researchers are building digital twins of the environment, security organizations predict migration patterns... There are many more examples.
Nobody expected Copernicus data to start such a revolution in this field. It was (and still is) a tremendous validation of ESA's operational capacity, but at the same time also significant pressure on the data distribution systems as they were not expecting such uptake. Still, the Copernicus Data Hubs have already distributed an order of magnitude more data than is published. With user uptake growing further, as well as the recognized importance of Earth Observation data for the monitoring of climate change, the EU decided to invest in the next level of user data processing and distribution infrastructure. The new Copernicus Data Space Ecosystem was born.
The future of European user data processing and distribution is powered by experienced players - T-Systems and CloudFerro, with their well-used cloud infrastructure, Sinergise and VITO with Sentinel Hub and OpenEO data discovery and processing tools, and DLR, ACRI-ST, and RHEA taking care of on-demand processing and Copernicus Contributing Missions.
What does it mean to CREODIAS users?
This is CloudFerro long-term commitment to grow and maintain the Copernicus EO data repository available in CREODIAS. Within the next few years, one of the largest online repositories worldwide will grow from 35PB to over 85 PB of free and immediately available EO data. Data from all over the world is available directly from the cloud through efficient, cloud-native access mechanisms (both API and GUI). Data is available for current users, new users, and federated platforms.
Copernicus Data Space Ecosystem - what’s in it for users?
- The data offer available for the user will increase. The data repository includes full up-to-date Copernicus data as well as complimentary commercial data offering (EO data+)
- Data that can be processed directly in a cloud environment
- Expanded search and visualisation of the data with Jupyter notebooks for testing and development
- Federation with other EO services – Sentinel Hub, OpenEO, JupyterHub, and others
- Serverless, on-demand processing services – for Sentinel products and other data
- Many services will be free of charge with easy access to a payable but easily scalable extension
Data offer
First and foremost, it's about the data we guarantee - instant data availability. Just about all the data ever acquired by Sentinel satellites, with minor exceptions, will be available online, instantaneously including e.g. Sentinel-1 SLC and GRD and L2 OCN, Sentinel-2 L1C, and L2A (reprocessed to Collection 1, as reprocessed data becomes gradually available), Sentinel-3 and Sentinel-5P L1 and L2 data as well as Copernicus Contributing Missions data. Full archive, and always up-to-date.
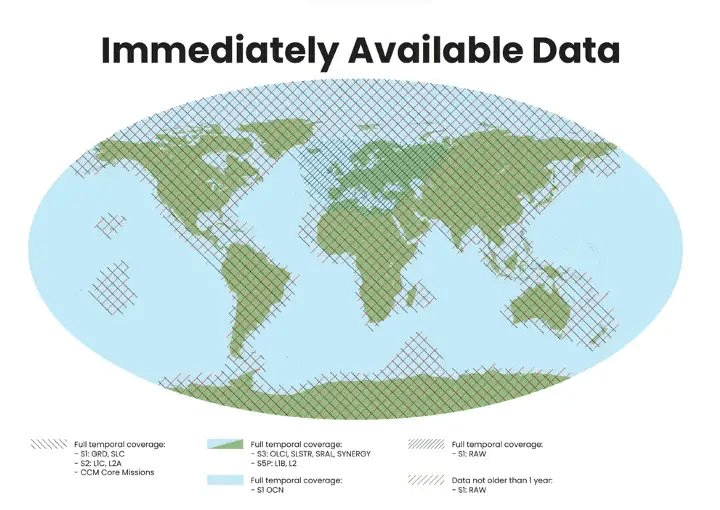
There is currently no infrastructure where all of this would be available in one place. The new Copernicus Data Space Ecosystem will be it. Data will be available through various interfaces – from old-fashioned download, direct S3 access with STAC items, and cloud-optimized formats to streamlined access APIs, which are able not just to fetch the data but also to process it.
EO services
There is a web-based application built on top of a very popular EO Browser technology to allow for data visualisation and as a user interface to access the data. Several on-demand processors are capable of building non-default formats and derived products, such as Sentinel-1 coherence and CARD4L products, MAJA-powered atmospheric processing, and similar. The data can be accessed via the openEO and JupyterHub for serverless processing. Last but not least, there will be a special focus put on traceability. For all data managed within the Copernicus Data Space Ecosystem, it will be possible to trace where it came from and what operations were performed on it.
Very important – the vast majority of these capabilities will be available free for use, funded by the European Union. The quotas designated for users should be more than sufficient for the individual's use - personal, research, or commercial. For those interested in the larger-scale operation, there will be practically unlimited resources available under commercial terms in CREODIAS allowing them to build applications on a world scale. Furthermore, there will be significant credits made available, in the form of extra free resources, to be used for research and pre-commercial exploitation opportunities.
In addition to that, users will be able to add and disseminate their collections and processors. What is more, there will be a set of additional services available for the CREODIAS users, such as streamlined access to data, and on-demand processing.
It is all about timing, responsibilities, and commitments
The initiative has quite an intense phase-in plan, in order to allow hundreds of thousands of existing users of the Copernicus Data Hubs to migrate their workflows to the new service. First, a limited but stable, roll-out has just happened (24th January 2023) with continuous upgrades over the upcoming months until full service will be made available by the end of June 2023.
This is a very challenging yet super exciting opportunity for everyone in the remote sensing community - from beginners to experts, from researchers, companies, and institutions, as well as individuals. Just about anyone can benefit from being aware of what is happening with our planet. And we should all take interest in it, and act. For the consortium partners, however, this is especially important - we were given an opportunity to build a new ecosystem for everyone to use. An ecosystem that strives to provide a significant upgrade over existing tools and services and is open to welcoming new partners and service providers in the future! This comes with huge responsibility towards everyone - not just to the ESA and European Commission, but for the worldwide EO community. We are confident we can execute it, so stay tuned for further information.
Discover Copernicus Data Space Ecosystem.
Read more in our news and case studies.
How can satellite remote sensing help analyse forests?
Forests cover 31 percent of the world's land surface, that's over 4 billion hectares[1]. According to the research published in Nature Climate Change in 2021, forests are a natural ’carbon sink’ that absorbs a net 7.6 billion metric tonnes of CO2 per year. The degradation of timberland around the world has prompted scientists not only to seek ways to halt these processes but also to develop objective diagnostic methods to assess the status and changes occurring in forests. After all, there is a lot at stake – as the United Nation's Global Forest Goals Report 2021 estimates, 1.6 billion people worldwide depend directly on forests for food, shelter, energy, medicines, and income[2].
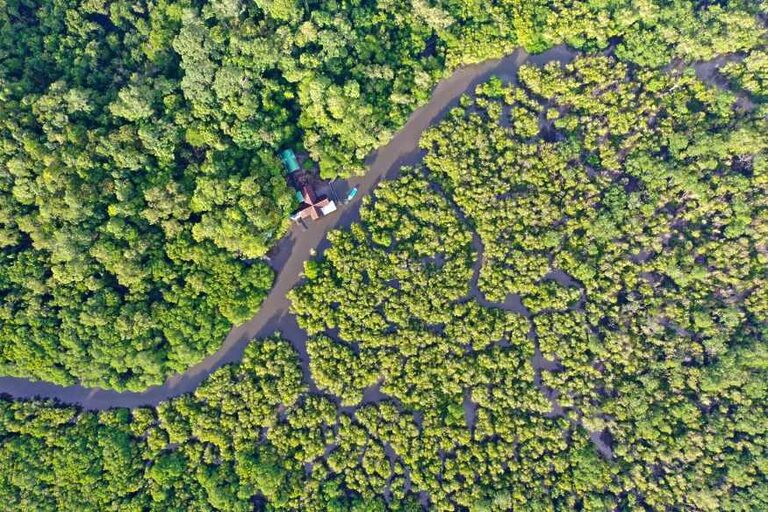
The development of technology and the integration of satellite data with environmental models have given us the ability to observe the Earth on a large scale. One of the tools used to monitor the state of the environment, especially complex ecosystems such as forests, is satellite remote sensing.
How satellites for remote sensing work
Satellite remote sensing includes a whole set of procedures involving the acquisition, processing, and interpretation of satellite images, from which spatial information is extracted. Images of a given area can be taken regularly with successive satellite overpasses, which makes it possible to observe changes that have occurred in each area even over a long period of time. These methods allow us to observe changes in land cover, analyse the condition of vegetation over large areas, or monitor the extent of natural disasters, such as droughts, floods, and others. Moreover, remote sensing methods effectively reduce costs and research time. The CREODIAS platform, for example, has more than 30 PB Copernicus EO data available free of charge and off-the-shelf. This platform and similar platforms allow for data to be processed in the cloud without the need for time-consuming downloads to local computers or arrays. Moreover, the public cloud for their processing that CloudFerro provides is a much more cost-effective solution than having infrastructure on one's own.
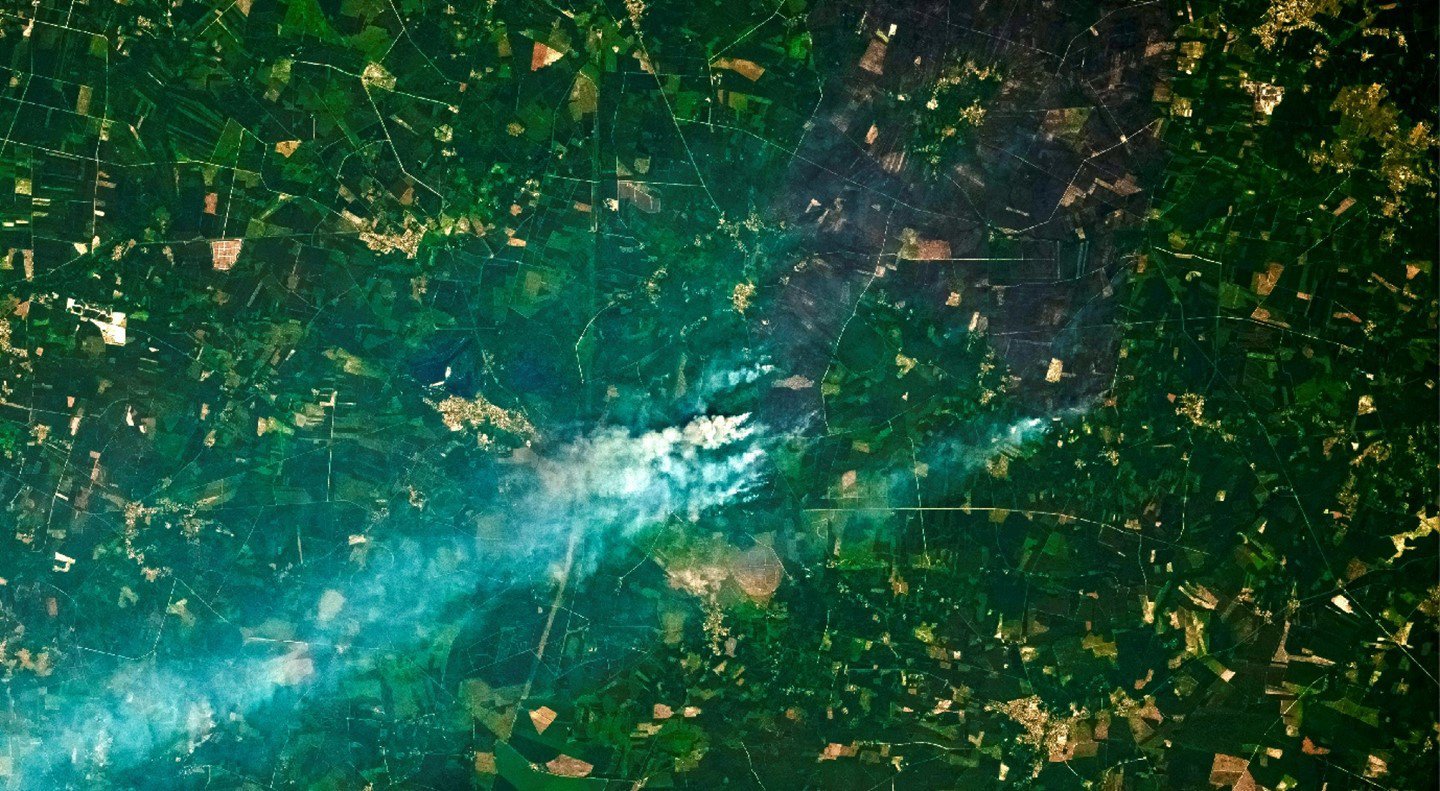
Observing forests from space
Applying remote sensing techniques to monitor such complex ecosystems as forests can be a laborious undertaking. The complexity lies mainly in the multi-layered structure of forests, their species composition, and spatial variability. Therefore, when analysing images from several months, we can see completely different objects, despite the fact that the characteristics of the forest have remained unchanged.
However, this can bring some advantages to the process. For instance, if we dispose of multi-temporal data, we can better classify the species because their evolution during the growing season is specific. The growing popularity of remote sensing can also be attributed to its detail and comprehensiveness. Today, satellites are able to capture high-resolution images, making it possible to detect even individual trees.
The process of forest area analysis
Today, remote sensing is mainly used for ongoing monitoring and forest inventories. A satellite image is a unique way to do it on a global scale. Even at regional, national, or local scales, remote sensing is often the only way to obtain data on forests located in hard-to-reach places or dangerous regions of the world. Such data can be used by local forest management institutions and global environmental agencies to monitor the condition of the area, and manage environmental emergencies.
The first stage of forest monitoring is determining its spatial extent, where different remote sensing data, are used. This information, combined with the documentation on land use, allows us to create accurate maps, but also to indicate areas of succession.
Knowing the extent of forests, satellite data can also be used for forest analysis to:
- determine the size (volume) of the forest, i.e., the amount of biomass present in a given area. This allows us to estimate the amount of wood that can be harvested, as well as determine the amount of carbon in the ecosystem
- rapidly and accurately identify changes on the forest surface caused by wind, fires, floods, or sustained or human activities
- to map all the impacts of human activities, even in the most remote areas of the planet.
Processing forest data in a cloud environment
One of the most advanced forest analyses performed on a global scale is Forest Digital Twin Earth Precursor developed by the European Space Agency, as part of the Digital Twin Earth Precursor (DTEP) initiative. Its aim is to create a digital replica of all the forest ecosystems, which involves integrating complex environmental models with a vast amount of Earth observation data combined with artificial intelligence solutions. CloudFerro, the operator of the CREODIAS platform, was a partner in the Precursor phase of the Digital Twin Earth project, delivering technical expertise and resources.
Large-scale research projects require advanced competencies and vast IT resources because they need to collect, store and process large volumes of data in an easy, cost-effective, and timely manner. CREODIAS’ powerful cloud infrastructure and its large repository of current and historic Earth observation data greatly facilitate conducting this type of research helping meet computation and storage requirements for processing growing volumes of data.
For more on species classification of forests, go to an article by a forest expert published on CREODIAS.
[1] FAO. The State of the World’s Forests 2022. Forest pathways for green recovery and building inclusive, resilient and sustainable economies. Rome, FAO (2022). https://doi.org/10.4060/cb9360en
[2] United Nations Department of Economic and Social Affairs, United Nations Forum on Forests Secretariat. The Global Forest Goals Report (2021). https://doi.org/10.18356/9789214030515